Enhancing the spatial resolution of satellite gravimetry model
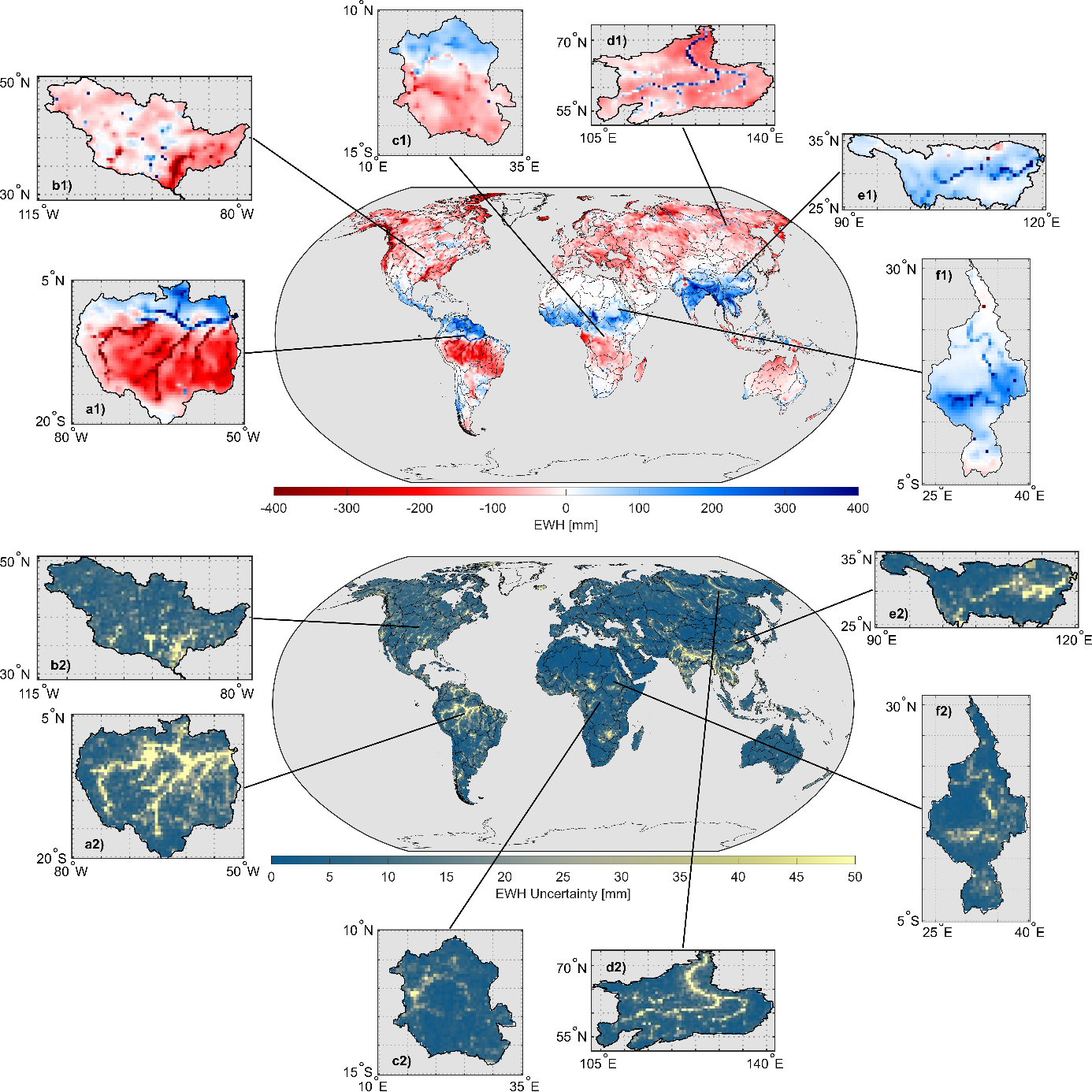
Since 2002, the Gravity Recovery and Climate Experiment (GRACE) and its follow-on mission have been providing us a unique view to study the monthly variations in the Earth’s gravity field. With their contribution, we can investigate the Earth’s water cycle from a macro perspective. One of the major challenges of the GRACE(-FO) mission is the coarse spatial resolution limited by measuring principles and postprocessing approaches. In this project, we employ deep learning algorithms to combine GRACE measurements and high-resolution simulations to downscale the GRACE products to the same spatial resolution as the high-resolution information.
Reference: Gou, J., Soja, B. Global high-resolution total water storage anomalies from self-supervised data assimilation using deep learning algorithms. Nature Water (2024). external page https://doi.org/10.1038/s44221-024-00194-w
Data: Gou, J., Soja, B. GRACE-SeDA: A global total water storage anomaly product with a spatial resolution of 0.5 degrees from self-supervised data assimilation. ETH Research Collection (2023); external page https://doi.org/10.3929/ethz-b-000648738
Contacts:
Junyang Gou, ETH Zurich,
Benedikt Soja, ETH Zurich,