Development of ML algorithms for geodesy
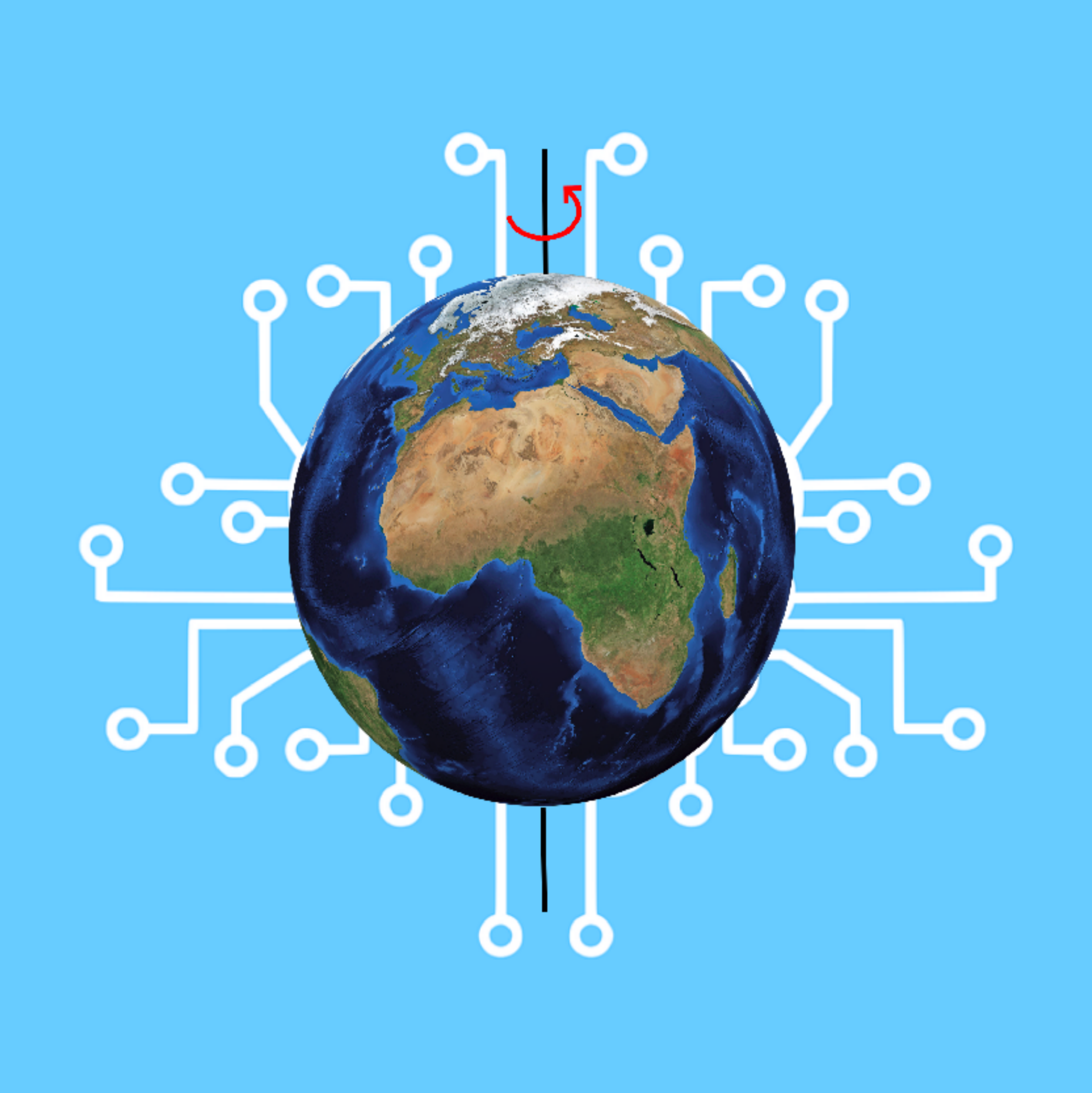
With the increasing volume of geodetic data, new mathematical models have the potential to exploit the data for scientific purposes. These mathematical models are based on big data processing algorithms that are typical in the fields of data science and machine learning. However, geodetic data have important physical interpretations and the mathematical methods should reflect that. From sequential models to graph neural networks, we develop new, physically informed machine learning algorithms to deal with various problems in geodesy. Additionally, we are focusing on the inclusion of observational errors in machine learning frameworks. Based on the theoretical results obtained, we analyze and predict GNSS and earth orientation parameters time series with state-of-the-art accuracy.
Contacts:
Mostafa Kiani Shahvandi, ETH Zurich,
Benedikt Soja, ETH Zurich,