New publication by Fuso et al. (2024)
"Machine learning-based detection of TEC signatures related to earthquakes and tsunamis: the 2015 Illapel case study" by Fuso et al. (2024)
The ionospheric Total Electron Content (TEC) can be perturbed by acoustic and gravity waves (AGWs) triggered by earthquakes and tsunamis. These are known as Travelling Ionospheric Disturbances (TIDs) and can be detected in real-time through the VARION (Variometric Approach for Real-Time Ionosphere Observation) algorithm.
In this study, we present a VARION-based machine learning model able to detect TEC perturbations, exemplified by the 2015 Illapel case study. Using the VARION-generated observations (i.e., dsTEC/dt) provided by 115 GNSS stations, we consider both Random Forest and XGBoost classifiers, achieving the best performance with the last one. The model reaches an F1 score of 0.77, recall of 0.74, and precision of 0.80 on the test data, presenting an average difference between the labeled and predicted middle epochs of TEC perturbation of 75 seconds. Finally, the model could be seamlessly integrated into a real-time early warning system, due to its low computational time.
Here you can find the article:
external pagehttps://link.springer.com/article/10.1007/s10291-024-01649-z
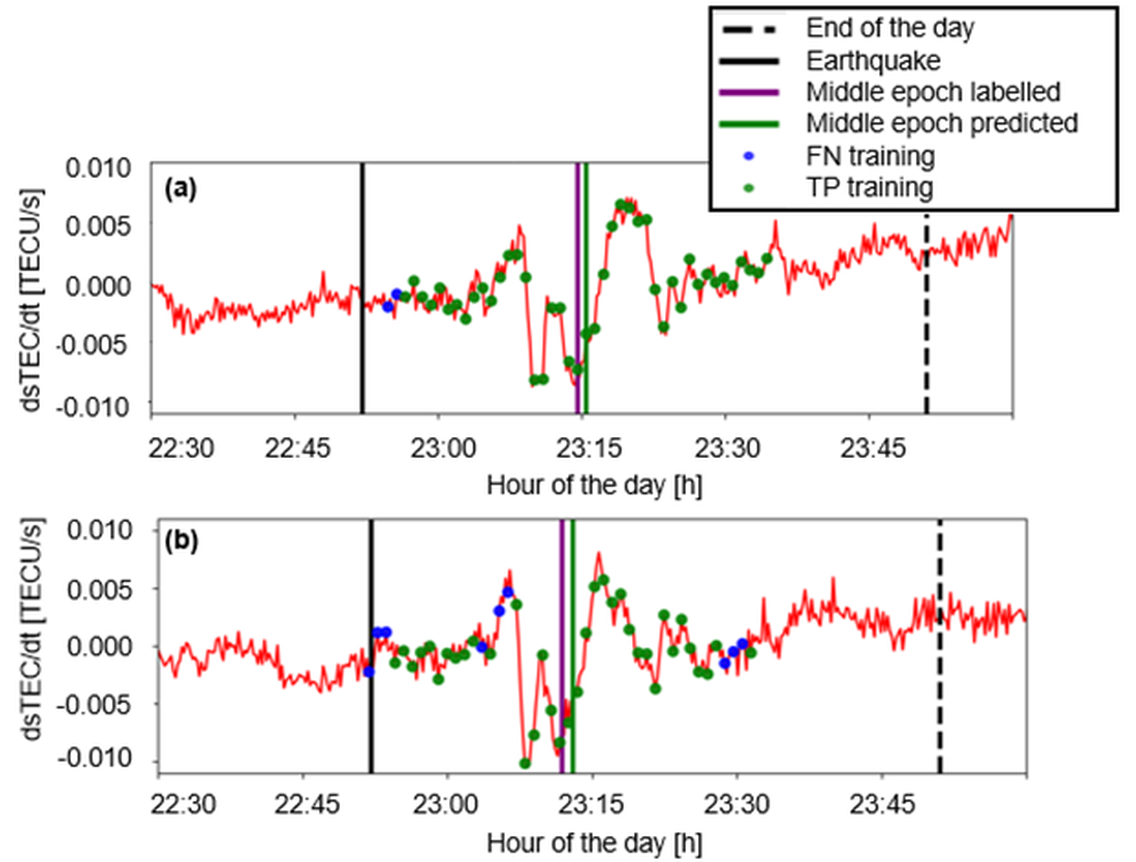