New publication by Pan et al. (2023)
“Machine learning-based multipath modeling in spatial domain applied to GNSS short baseline processing” by Pan et al. (2023)
Multipath is the main unmodeled error source hindering high-precision GNSS data processing. Conventional multipath mitigation methods, such as sidereal filtering (SF) and multipath hemispherical map (MHM), have certain disadvantages: They are either not easy to use or not effective enough for multipath mitigation.
Pan et al. formulated multipath modeling as a regression task using machine learning (ML), and multipath was fitted with respect to azimuth and elevation. The performance of the proposed method was evaluated with 1 s and 30 s sampling rate GPS data, respectively. It confirms that the ML-based method outperforms SF and MHM and achieves the highest residual reduction rates. In conclusion, the ML-based multipath mitigation method is effective, easy to use, and can be easily extended by adding auxiliary input features during model training.
Find the full article here:
external page https://doi.org/10.1007/s10291-023-01553-y
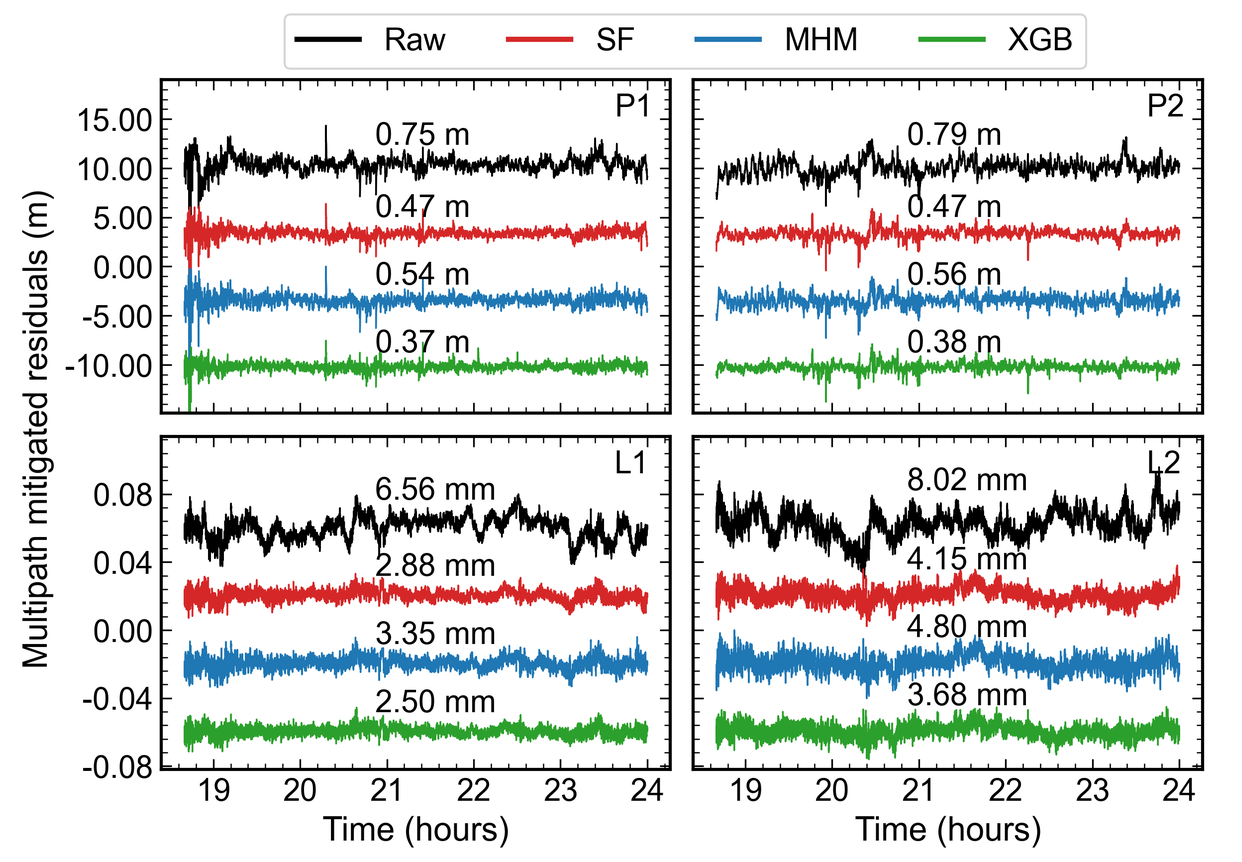